OpenCRISPR-1: Generative AI Meets CRISPR
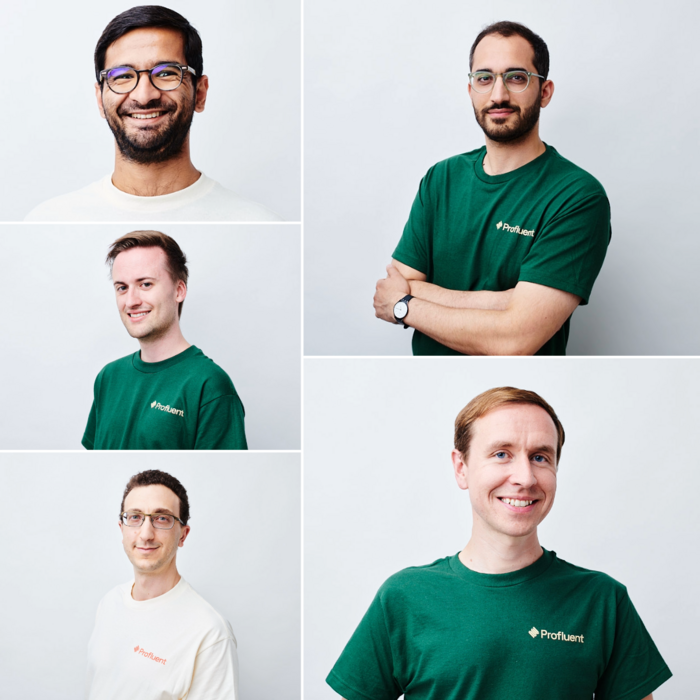
In a recent study, researchers at Profluent Bio (Berkeley, CA, USA) used artificial intelligence (AI) to design novel CRISPR gene editors that are functional and that show comparable or improved genome-editing activity and specificity relative to naturally occurring gene editors, despite being hundreds of mutations away from any known natural protein. This innovative approach has yielded OpenCRISPR-1, the first AI-generated gene editor.
In a proof-of-concept study, OpenCRISPR-1 demonstrated comparable efficiency to the widely used Streptococcus pyogenes Cas9 (SpCas9) while offering improved specificity. This development not only expands the CRISPR toolbox but also paves the way for creating gene editors tailored to specific applications, which could range from agriculture to medicine.
»Our LLMs, trained on billions of proteins in nature, are able to learn the sequence-to-function mapping of natural proteins and can be utilised to build functional proteins from scratch, such as OpenCRISPR-1,« said Ali Madani, PhD, founder and CEO of Profluent Bio and senior author of the study.
»We can now use the model as a guide to install additional desired features, for example, a set of mutations that alter PAM selectivity, a combination of deletions to reduce size, or a set of mutations to alter thermostability while simultaneously maintaining other key properties, such as processivity and stability,« he added. »This can be very difficult to achieve with directed evolution because the addition of one property may impair another. AI can circumvent this.«
The study has not been peer-reviewed and is available as a preprint on bioRxiv.
Rationale: Addressing limitations of natural CRISPR-Cas systems
CRISPR-Cas systems, originally evolved as bacterial defence mechanisms against viruses, have been repurposed as powerful gene-editing tools. However, these natural systems often face limitations when applied in non-native environments, such as human cells.
Traditional approaches to optimising these tools for use in non-native environments include directed evolution and structure-guided mutagenesis. Although these approaches have yielded improvements, they are limited by the need for explicit structural hypotheses or complex screening processes.
Directed evolution, for instance, can be limited by the complex nature of ‘fitness landscape’, a way to visualise how different genetic variations perform. On the other hand, structure-guided approaches depend on solved structures representing key functional states that are often difficult to obtain for complex functions beyond simple binding interactions.
The objective of this study was to edit the human genome for the first time using a gene editor that was designed entirely using AI. »This was a scientific moon-shot, as CRISPR-Cas proteins are incredibly complex molecular machines with precise functions that require an understanding of protein-protein as well as protein-nucleic acid interactions,« said Dr. Madani.
Approach: Using AI to design novel, optimised editors
To overcome the limitations of traditional approaches for optimising gene editors, the research team leveraged the power of large language models (LLMs) trained on vast amounts of biological data to generate novel CRISPR-Cas proteins that could function as efficient gene editors in human cells.
To this end, the team compiled the CRISPR-Cas Atlas, an extensive dataset of over one million CRISPR operons from diverse microbial genomes, by mining 26 terabases of assembled genomes and metagenomes.
»To our knowledge, CRISPR-Cas Atlas is the most extensive dataset of CRISPR systems curated to date,« emphasised Dr. Madani.
Using this dataset, the researchers fine-tuned ProGen2, a protein language model previously developed by Profluent, to specialise in generating CRISPR-Cas proteins. The team balanced the training data for protein family representation and sequence cluster size to ensure broad coverage. The fine-tuned models were used to generate four million novel CRISPR-Cas protein sequences. Half were generated directly, while the other half were prompted with short segments from natural proteins to guide generation towards specific families.
“Typical bioinformatic mining efforts in industry are directed toward searching for an ideal system in nature, akin to finding a needle in a haystack. Our atlas was curated with the primary objective of feeding as much data as possible to our LLMs to learn the underlying language and biophysical associations behind CRISPR systems. Once our LLMs gain mastery of this language, we can generate sequences across CRISPR-associated families as desired”Ali Madani, PhD, CEO and Founder of Profluent Bio
According to Dr. Madani, fine-tuning ProGen2 using the CRISPR-Cas Atlas enabled the model to benefit from a general representation of proteins across the diversity of life and a tailored understanding of CRISPR-associated systems.
Commenting on the novelty of this approach, Dr. Madani said: »Typical bioinformatic mining efforts in industry are directed toward searching for an ideal system in nature, akin to finding a needle in a haystack. Our atlas was curated with the primary objective of feeding as much data as possible to our LLMs to learn the underlying language and biophysical associations behind CRISPR systems. Once our LLMs gain mastery of this language, we can generate sequences across CRISPR-associated families as desired.«
»LLMs can analyse vast amounts of biological data, learning patterns and relationships that are not immediately obvious. This allows for the generation of novel, functional CRISPR-Cas variants with high efficiency and accuracy, potentially uncovering designs that might be missed by traditional techniques,« said Prof. Manuel Kaulich, PhD (Goethe University Frankfurt), who was not involved in the study. “LLMs are novel and outpace traditional methods in efficiency, scalability, and automation.”
Generated sequences were filtered and clustered to assess novelty and diversity. To evaluate structural viability, the researchers used AlphaFold2 to predict the structures of 5,000 AI-generated sequences. A subset of 209 generated Cas9-like proteins were synthesised and tested for gene-editing activity in human cells for experimental validation. The researchers assessed both on-target efficiency and off-target effects across multiple genomic sites using next-generation sequencing.
AI expands CRISPR-Cas diversity
The CRISPR-Cas Atlas included over one million CRISPR operons, offering 2.7 times more protein clusters across all Cas families compared to UniProt. The sequences generated by the LLM represented a 4.8-fold expansion of diversity compared to natural CRISPR-Cas proteins, with even greater expansions for families with few natural proteins, such as Cas13 (8.4-fold expansion) and Cas12a (6.2-fold expansion) (Figure 1).
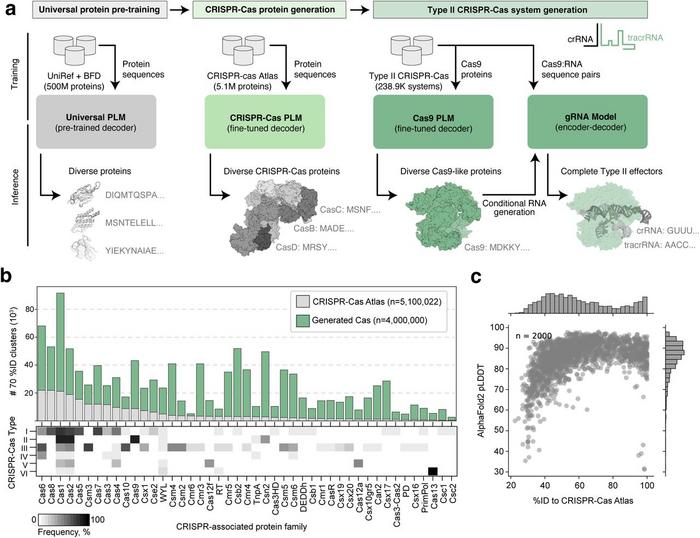
Despite significant sequence divergence from natural proteins (often with only 40-60% identity), the generated sequences were predicted to adopt folds highly similar to their natural counterparts, suggesting functional viability (Figure 1). Importantly, core Cas9 domains, such as the HNH and RuvC nuclease domains, PAM-interacting domain, and target recognition (REC) lobe, were present in most generated proteins at rates similar to those of natural sequences.
According to Dr. Madani, these findings suggest that LLMs can generate diverse, functional CRISPR-Cas proteins, bypassing evolutionary constraints. »Increasing the diversity of CRISPR-like proteins and expanding virtually all known CRISPR families beyond what exists in nature increases our ability to design bespoke gene editors for each application,« he said.
Dr. Madani added that it is highly unlikely that there is a Cas protein that has naturally evolved to have perfect PAM selectivity, catalytic efficiency, size, stability, and specificity to fix a particular mutation. This approach could help in the design of editors to fix the underlying causative mutations of various genetic diseases.
»AI can be used to interpolate between and extrapolate beyond what nature has explored, all the while with knobs at our disposal to optimise multiple desired properties simultaneously,« he said.
OpenCRISPR-1: A functional, highly specific AI-designed gene editor
The team selected 209 Cas9-like proteins for experimental characterisation. These were human codon-optimised, cloned into expression plasmids, and tested for gene-editing activity in HEK293T cells. Of the 209 tested Cas9-like proteins, many showed editing activity in human cells, with some performing on par with or better than SpCas9 (Figure 2).
»This study marks a major leap in the field by not just optimising existing proteins but creating entirely new variants with potentially superior characteristics. The generation of novel Cas proteins with enhanced activity and specificity has the potential to overcome some of the limitations of current CRISPR systems, such as off-target effects, limited target range, and large molecular weight,« noted Prof. Kaulich. »This could lead to more effective therapies for genetic diseases and improved tools for research.«
Furthermore, receiver operating characteristic (ROC) analysis — a method for assessing model performance or discriminate ability — showed that language model scores were highly predictive of enzyme activity, separating active and inactive enzymes with an area under the curve (AUC) value of 0.82.
»This is the first demonstration in human cells that AI can generate a functional gene editor from scratch. This is one solution among the millions that we can create from scratch,« highlighted Dr. Madani.
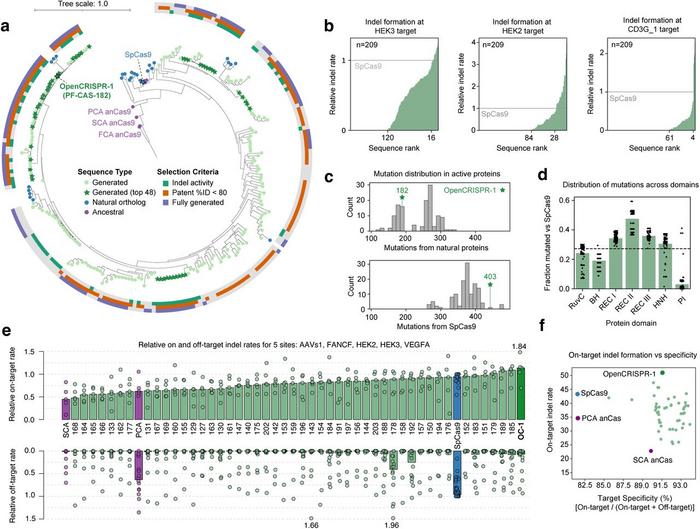
OpenCRISPR-1, the top performer among the 209 tested Cas9-like proteins, demonstrated comparable on-target editing efficiency to SpCas9 (median indel rates of 55.7% versus 48.3%), with a 95% reduction in off-target editing across multiple genomic sites tested (median indel rates of 0.32% versus 6.1%) (Figure 3).
»This high specificity is reminiscent of high-fidelity SpCas9 variants that have been described in the literature,« noted Dr. Madani.
When asked about potential explanations for the high specificity of OpenCRISPR-1, he said: »One hypothesis is that OpenCRISPR-1 may have altered kinetics and off-rates. We are aiming to test OpenCRISPR-1 across a larger panel of cell types and delivery methods to further explore potential trade-offs in activity and specificity.«
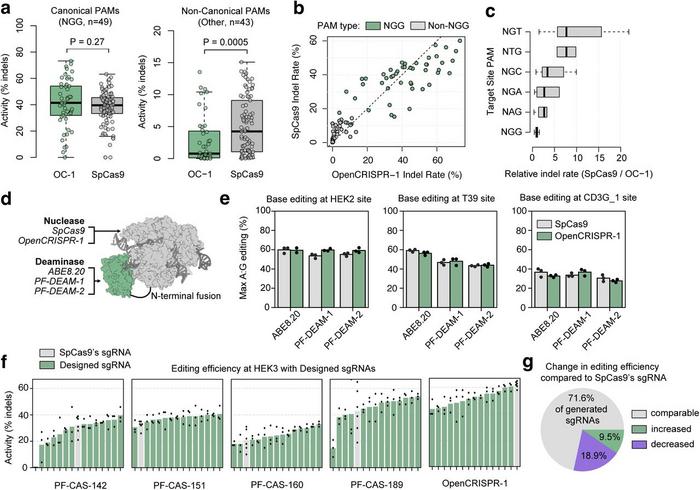
OpenCRISPR-1 is 1,380 amino acids long, with 403 mutations compared to SpCas9 and 182 mutations from any natural protein in the CRISPR-Cas Atlas. Moreover, OpenCRISPR-1 lacks immunodominant and subdominant T cell epitopes for HLA-A*02:01 that were previously identified in SpCas9, suggesting a potentially low immunogenicity for the AI-designed editor.
The authors have publicly released the OpenCRISPR-1 sequence to enable its broad usage across research applications.
Commenting on their rationale for making the sequence of OpenCRISPR-1 publicly available, Dr. Madani said: »Our goal in open-sourcing OpenCRISPR-1 is to further democratise gene editing. We encourage the use of AI for ethical research and commercial use, particularly in the development of medicines leveraging CRISPR, the groundbreaking scientific discovery that is being used in the development of new treatments for countless diseases. We’re excited to hear from the broader scientific community regarding feedback on OpenCRISPR-1 and how it compares with existing tools.«
Base editing and guide RNA modelling
To expand the potential applications of OpenCRISPR-1, the team converted the enzyme into a nickase and fused it with adenine deaminases, including the established ABE8.20 and novel AI-generated deaminases. OpenCRISPR-1 nickase showed robust A-to-G conversion rates (35%–60%) across multiple genomic loci (Figure 3). These conversion rates are similar to those achieved with previously established base editor systems, such as ABE8.20, PF-DEAM-1, and PF-DEAM-2.
»We have seen some early signs that the performance of base editors that are generated from scratch with AI may have favourable on-target and by-stander off-target profiles,« noted Dr. Madani.
The researchers also developed a sequence-to-sequence gRNA model to generate optimised single-guide RNAs (sgRNAs) for the AI-designed Cas proteins. The model-designed gRNAs were found to be similar to naturally derived gRNAs and could accurately predict the compatibility of sgRNAs between diverse Cas9 orthologs. Testing of these sgRNAs showed enhanced editing efficiency for several AI-generated Cas9-like proteins (Figure 3).
Looking ahead
According to Dr. Madani, this work represents the first successful precision editing of the human genome using an entirely AI-generated CRISPR enzyme and its associated RNA components. Although this represents a significant step forward in the development of engineered CRISPR-Cas proteins with optimised properties, the study primarily focused on Cas9-like proteins. Future work could expand to other CRISPR-Cas proteins, including Cas12 and Cas13, and explore more diverse functionalities.
»Rather than viewing OpenCRISPR-1 as a static asset, we are enthusiastically receptive to feedback on how it performs in multiple settings in the hands of the broader community. There is a lot of room for improvement, pivots, and growth,« Dr. Madani said.
“By enabling the rapid design of novel CRISPR-Cas systems with improved specificity and activity, this method paves the way for more precise gene therapies, potentially reducing off-target effects and improving patient outcomes. The approach could accelerate the development of treatments for genetic disorders, enhance research capabilities in synthetic biology, and enable the discovery of new therapeutic strategies”Manuel Kaulich, PhD (Goethe University Frankfurt)
Moreover, the study did not assess the long-term effects or potential immunogenicity of the AI-generated proteins, which is crucial for evaluating the potential use of AI-generated CRISPR systems for therapeutic applications. Furthermore, future studies are needed to further characterise and optimise OpenCRISPR-1 for potential therapeutic applications. Aspects of improvement include the enzyme’s activity, specificity, PAM selectivity, stability, and size.
Prof Kaulich commented on the potential of AI-designed CRISPR proteins for developing new gene therapies: »By enabling the rapid design of novel CRISPR-Cas systems with improved specificity and activity, this method paves the way for more precise gene therapies, potentially reducing off-target effects and improving patient outcomes. The approach could accelerate the development of treatments for genetic disorders, enhance research capabilities in synthetic biology, and enable the discovery of new therapeutic strategies.«
He noted, however, that the use of AI to generate gene-editing tools may raise ethical concerns and exacerbate inequalities if access to these technologies is limited to certain groups. »Ensuring the safety, accuracy, and reliability of AI-generated tools is crucial, requiring thorough experimental validation and robust regulatory oversight,« Prof Kaulich said. He added that addressing these concerns involves establishing clear ethical guidelines, promoting transparency, and ensuring equitable access to the technology.
Dr. Madani emphasised that OpenCRISPR-1 is only the first milestone in a long journey to cure disease. »We view AI-designed gene editors as an important tool that allows us to shift the drug development paradigm away from accidental discovery or limited manual engineering and toward intentional and rapid design of bespoke gene-editing solutions. Our hope is that, by lowering the costs and barriers to entry for therapeutic applications, AI-designed gene editors will accelerate innovation in genetic medicines.«
Link to the preprint:
Design of highly functional genome editors by modeling the universe of CRISPR-Cas sequences
Christos Evangelou, PhD, is a freelance medical writer and science communications consultant.