New Tool Offers Better Prediction of gRNA on-Target Efficiency
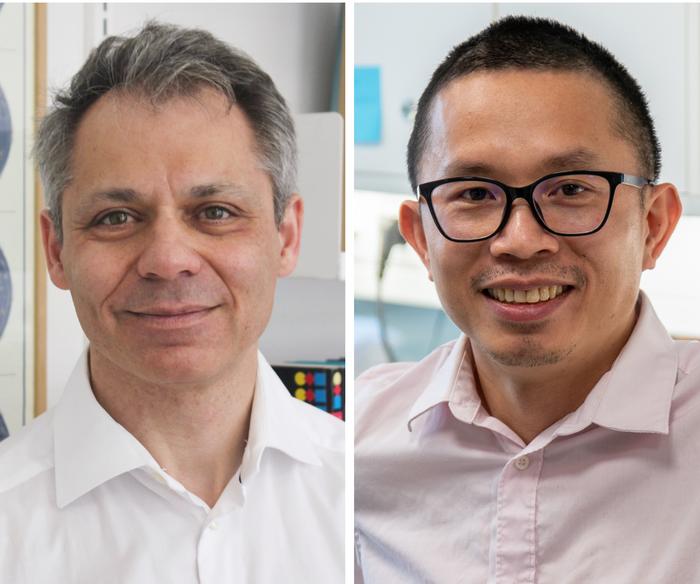
Accurate prediction of gRNA on-target efficiency is critical for the CRISPR workflow, and several deep learning models have been established to do this. Large datasets of known gene-editing activity of a gRNA on its corresponding endogenous site are needed to train such models, and this data can be hard to obtain.
A new approach packs the barcoded gRNA and its surrogate endogenous site into the same lentiviral vector. In a single experiment, thousands of different vectors can be used for the transfection of human cells. Gene-editing events for each of the gRNAs can then be studied by sequencing amplicons generated from simultaneous amplification in a mixed pool of transfected cells using a common primer set.
»This approach has allowed us to generate on-target efficiency data for more than 10,000 gRNAs. We used this data and included data from around 13,000 other previously published gRNAs to train a deep learning model, CRISPRon. We find that CRISPRon is significantly better at predicting gRNA efficiency than existing models,« says Yonglun Luo, who is co-senior author of the study that was published last Friday in Nature Communications. Luo is a molecular biologist and associate professor at the Department of Biomedicine, Aarhus University, Denmark.
“We have tested CRISPRon against the best methods out there, and we are consistently better. So I would say we have the best model”Jan Gorodkin
The other co-senior author, Jan Gorodkin, is a bioinformatician and professor at the Center for non-coding RNA in Technology and Health, IVH, University of Copenhagen, Denmark. He adds that most existing models are based on a limited number of gRNA activity data:
»The short story is that more data gives better performance. Moreover, methodological improvements contribute as well, for example, when our input includes the effective binding energy, ΔGB, of how strongly the gRNA interacts with the target DNA. Nobody has considered this before, and we find that it improves the performance of our model.«
Algorithms are trained to spot the most efficient gRNAs
Anyone who works with CRISPR knows that there is always a difference from gRNA to gRNA. Some work more efficiently and yield a high frequency of intended edits, while others are less efficient. Many of these differences are not obvious and are impossible to predict by just looking at the sequences, so over the past few years, there have been many efforts to develop models that can help scientists select the most efficient gRNAs.
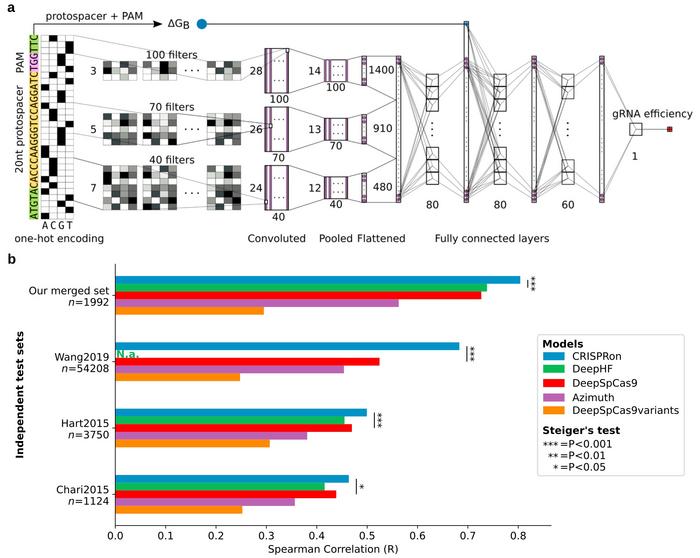
These models are often based on machine learning or deep learning using algorithms that find a pattern in large datasets of gRNAs and their known efficiency to edit a specific target sequence. The algorithms are trained with data for which the efficiency of each gRNA is known, and they learn how to predict the correct efficiency for new gRNAs they have not been presented with before. Importantly, the trained method has to be tested on independent data not used for the training.
CRISPRon was tested on several such data sets, and the team compared the results to a range of other methods including four best-in-class gRNA prediction models. Not only had CRISPRon the best prediction outcome, but the team’s own test dataset also had higher quality than the datasets used to train the other models.
»We have tested CRISPRon against the best methods out there, and we are consistently better. So I would say we have the best model,« says Gorodkin and adds:
»PhD student Giulia Corsi and Dr. Christian Anthon have done amazing work in obtaining the model and accompanying webserver.«
Also to be acknowledged is the group of molecular biologists that contributed to data acquisition, many of whom come from the Lars Bolund Institute of Regenerative Medicine in BGI-Shenzhen, China, where Luo is also affiliated.
A lentiviral library allows for massive quantification of gRNA efficiency
To obtain the high-quality data used to train CRISPRon, the team started with a selection of around 3,800 genes that have the potential to be targeted for drug development, e.g., receptors and kinases. The team then used computer algorithms to find 12,000 potential gRNAs targeting exons in these genes in compliance with a set of rules that included a nearby PAM “NGG” sequence and a limited number of predicted off-target sites.
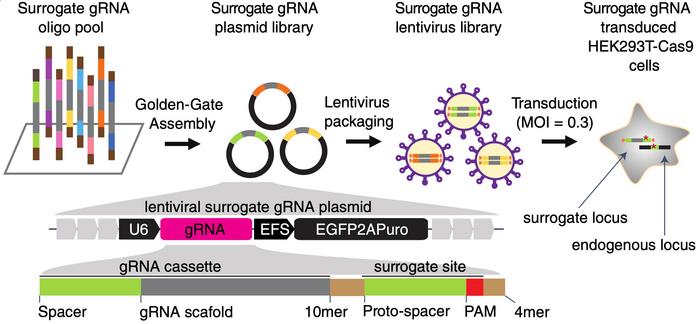
»The whole method is based on a lentiviral library, where each lentivirus encodes one of these gRNAs. Most importantly, they also encode a surrogate target site. The surrogate site is identical to the endogenous chromosomal sequence that the gRNA targets, and it includes protospacer and PAM sequences,« says Luo.
He explains that when HEK293T cells expressing Cas9 are transfected with the lentivirus, Cas9 may cut both the surrogate site and the endogenous site. The team subsequently looked for indels in the surrogate site and assumed that this would reflect what had happened at the endogenous site.
“So this is the magic method we have developed to generate the gRNA efficiency”Yonglun Luo
»If you wanted to examine the endogenous sites, you would have to use specific primers for each of them, and as you can imagine you can't do that for 12,000 sites. But since the surrogate sites are all in the same lentiviral vector, we can use one primer set to amplify all 12,000 sites simultaneously in the cells in a single PCR reaction. So this is the magic method we have developed to generate the gRNA efficiency,« Luo explains.
He notes that they also examined indel frequencies at several endogenous sites and found that the efficiency between them and the corresponding surrogate sites was quite well correlated.
Luo points out that the approach has been used before by other researchers but that his team has optimised the method to make the experimental procedure more efficient and straightforward. Among these modifications are the inclusion of eGFP and puromycin resistance genes in the lentiviral vector, which allows for better enrichment of the CRISPR-edited cells after transfection.
CRISPRon is publicly available on the web
The name of the new model for predicting gRNA efficiencies, CRISPRon, should not be confused with other techniques bearing the same name, which enable conditional activation of the CRISPR-Cas9 complex. The suffix ‘on’ refers to on-target gRNA efficiency, and back in 2018, Gorodkin headed the development of a model for predicting gRNA off-target activity, called CRISPRoff.
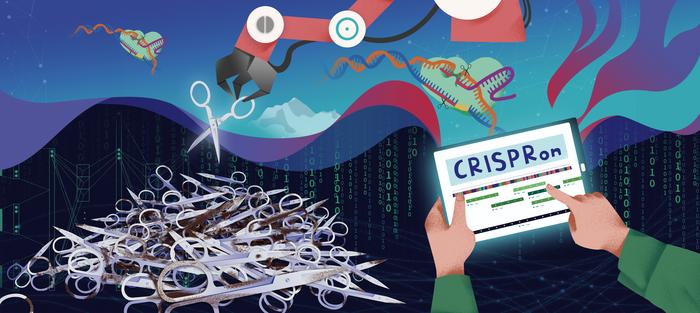
»We have made both models - CRISPRon and CRISPRoff - publicly available on our website. Here you can enter a chromosomal region or gene name, and about a minute later the model will tell you what the good gRNAs are,« says Gorodkin. With the graphical output you can quickly see how the gRNAs align to the sequence, and it also lists their efficiencies based on the predicted indel frequencies. You can also download the output and work with it on your own computer.
The team is now working to integrate the two models further, and they hope that their new and more accurate tool for gRNA efficiency predictions will help the scientific community.
Link to the original article in Nature Communications:
Enhancing CRISPR-Cas9 gRNA efficiency prediction by data integration and deep learning
The CRISPRon and CRISPRoff models are both publicly available on https://rth.dk/resources/crispr/
Tags
CLINICAL TRIALS
Sponsors:
Suzhou Maximum Bio-tech Co., Ltd.
Sponsors:
Zhejiang University